The recent tremendous success of unsupervised word embeddings in a multitude of applications raises the obvious question if similar methods could be derived to improve embeddings (i.e. semantic representations) of word sequences as well. We present a simple but efficient unsupervised objective to train distributed representations of sentences. Our method outperforms the state-of-the-art unsupervised models on most benchmark tasks, highlighting the robustness of the produced general-purpose sentence embeddings.
By Matteo Pagliardini, Prakhar Gupta, Martin Jaggi
Codes and Models are available at:
https://github.com/epfml/sent2vec
https://arxiv.org/abs/1703.02507
More from our feed
New Article Explores the Transformative Intersection of Generative AI and Intellectual Property
Read more
Join us at IP Service World in Munich!
Read more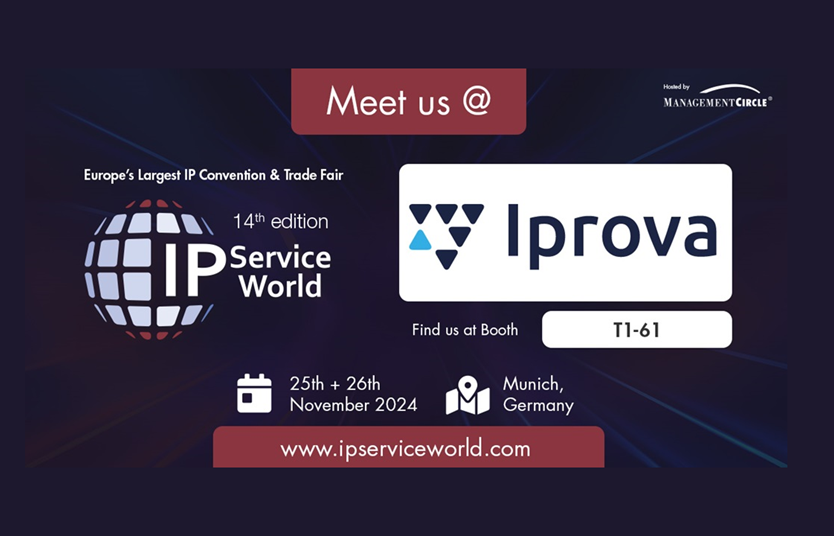
Webinar: AI-Assisted Invention – Results, Technology and Organisational Impact
Read more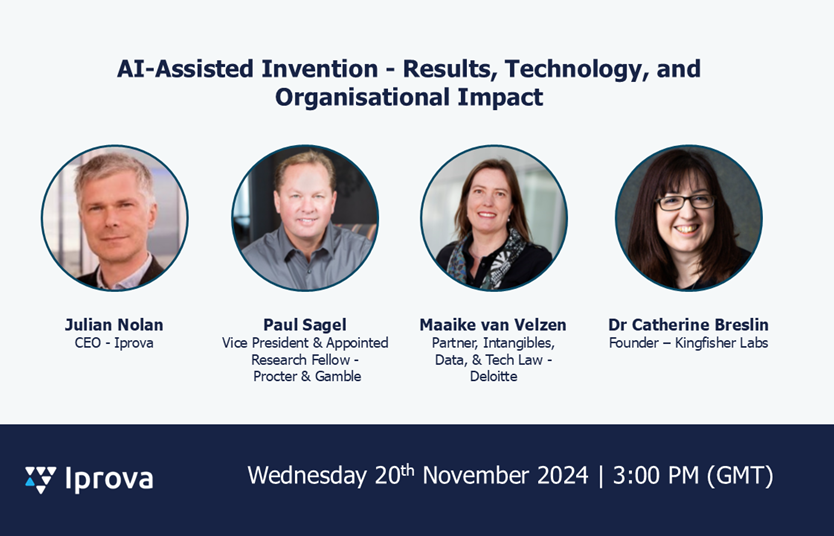